Data driven mechanics
The emergence of additive manufacturing techniques has enabled the creation of complex 3D shapes with topological feature sizes spanning length scales from nanometres upwards. These manufacturing technologies have facilitated the creation of new materials (metamaterials) with previously unattainable properties such as light and recoverable ceramics.
Using state-of-the-art advances in visualization and data assimilation, we obtain billions of high fidelity point cloud datasets in terms of measured defect distributions in the printed specimens including cracks and voids, as well as deformations (strains) at every material point via Digital Volume Correlation (DVC) on spatio-temporal XCT scans. Employing such extensive in-house data, we leverage recent foundational advances in machine learning and graph neural networks (GNNs), to design new additively manufactured materials with fully tailorable mechanical properties.
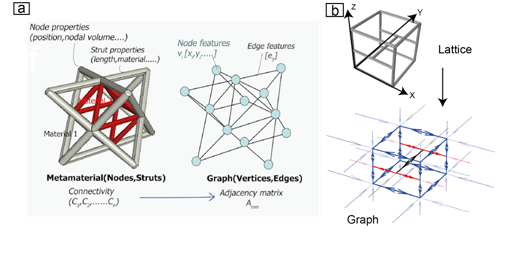
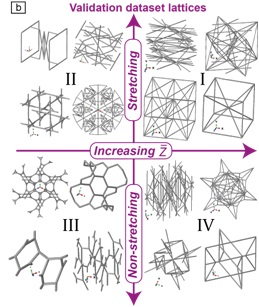
Key Literature
Indurkar, P. P., Karlapati, S., Shaikeea, A. J. D., & Deshpande, V. S. (2022), Predicting deformation mechanisms in architected metamaterials using GNN, arXiv:2202.09427.
Wang, Z., Joshi, A., Shaikeea, A. J.D. and Deshpande, V.S. (2023). Non-Speckle-based DVC for Measuring Large Deformations in Homogeneous Solids using a Laboratory X-ray CT. submitted_version